Machine Learning in Supply Chain Management: Optimization and Efficiency
5 min read
23 Sep 2024

Machine Learning (ML) is revolutionizing supply chain management by optimizing operations, enhancing efficiency, and improving decision-making processes. This article explores the transformative impact of ML in supply chain management, from demand forecasting and inventory optimization to logistics planning and predictive maintenance.
Demand Forecasting and Inventory Optimization
ML algorithms analyze historical sales data, market trends, and external factors to predict future demand accurately. Demand forecasting models use regression, time series analysis, and neural networks to anticipate customer preferences, seasonal variations, and supply chain disruptions. By optimizing inventory levels and replenishment strategies, ML-driven forecasts minimize stockouts, reduce excess inventory costs, and improve inventory turnover rates, ensuring efficient supply chain operations and customer satisfaction.

Logistics and Route Optimization
ML enhances logistics management by optimizing route planning, fleet management, and transportation scheduling. Predictive analytics algorithms optimize delivery routes, consider traffic patterns, weather conditions, and delivery constraints to minimize transportation costs and improve on-time deliveries. ML-powered logistics platforms automate decision-making processes, adapt to real-time changes, and enhance supply chain agility, enabling businesses to respond swiftly to market demands and logistical challenges.
Supplier Relationship Management
ML improves supplier relationship management (SRM) by analyzing supplier performance data, evaluating vendor reliability, and predicting supplier risks. Supplier segmentation models classify vendors based on performance metrics, delivery reliability, and quality standards. ML-driven SRM systems automate vendor selection, negotiate contract terms, and optimize procurement processes, fostering strategic partnerships and ensuring supply chain resilience and continuity.
Predictive Maintenance and Asset Management
ML algorithms enable predictive maintenance by analyzing sensor data, equipment performance metrics, and failure patterns. Predictive maintenance models detect anomalies, predict equipment failures, and recommend proactive maintenance interventions to minimize downtime and optimize asset utilization. ML-driven asset management systems extend equipment lifespan, reduce maintenance costs, and enhance operational efficiency across manufacturing, warehousing, and distribution facilities.
Challenges and Implementation Considerations
Implementing ML in supply chain management presents challenges such as data integration complexities, algorithmic bias in decision-making, and organizational readiness for digital transformation. Overcoming these challenges requires robust data governance frameworks, cross-functional collaboration, and investment in talent with expertise in data science and supply chain analytics. Addressing ethical considerations and ensuring transparency in AI-driven decision-making are essential to building trust and achieving sustainable benefits from ML technologies in supply chain operations.
Future Directions
The future of ML in supply chain management will likely see advancements in autonomous logistics, blockchain integration, and AI-driven risk management. Innovations in ML-powered demand sensing, real-time supply chain visibility, and sustainable supply chain practices aim to enhance supply chain resilience, optimize resource allocation, and mitigate operational risks in global supply networks. ML's role in supply chain management continues to evolve, offering opportunities to drive innovation, achieve operational excellence, and deliver superior customer value in competitive markets.
In conclusion, ML is reshaping supply chain management by optimizing operations, enhancing efficiency, and mitigating risks through advanced analytics and predictive capabilities. By harnessing ML technologies strategically and ethically, businesses can streamline supply chain processes, improve decision-making accuracy, and adapt to dynamic market conditions with agility and resilience.
More Articles
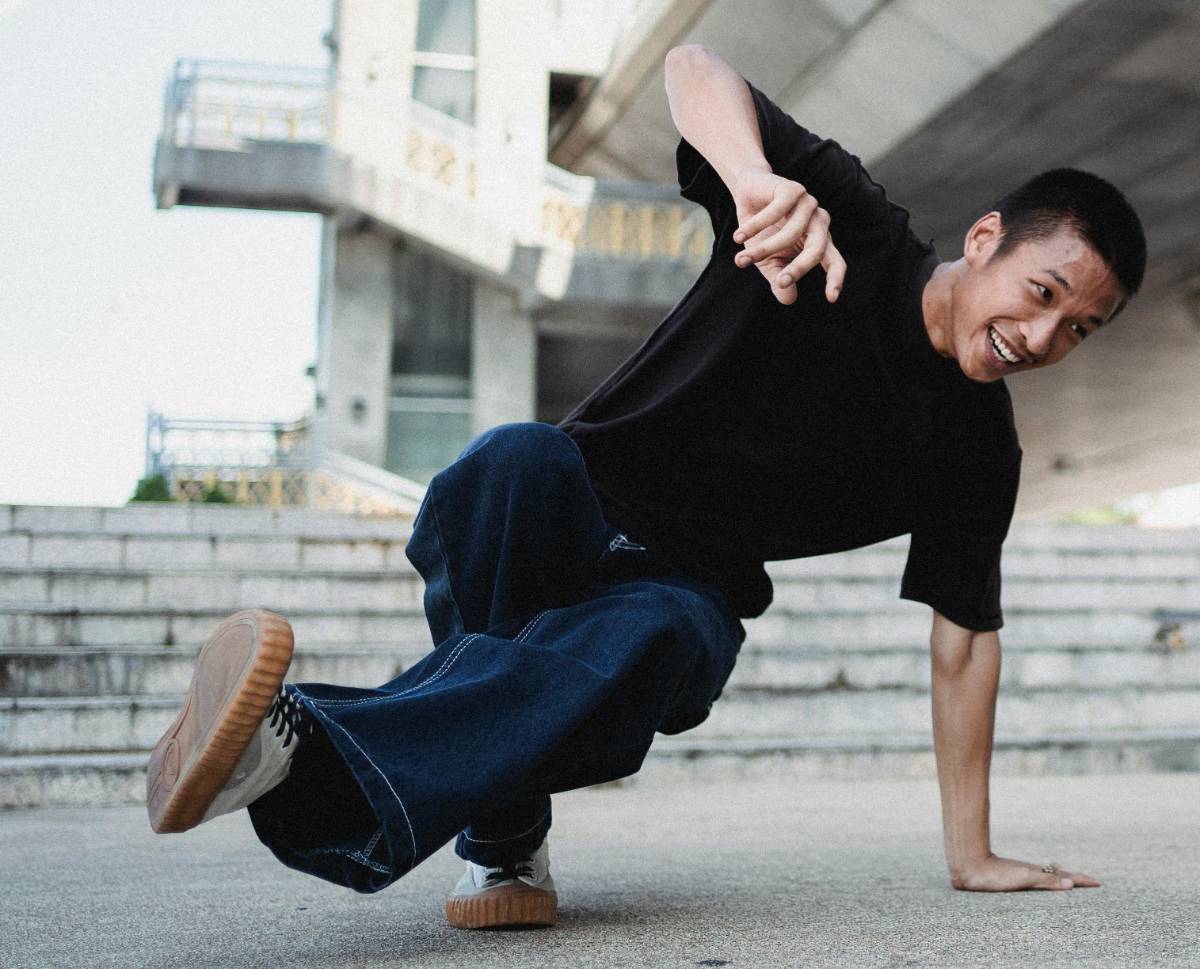
The Metaverse Beckons: How Blockchain Will Shape Virtual Worlds
6 min read | 03 Sep 2024
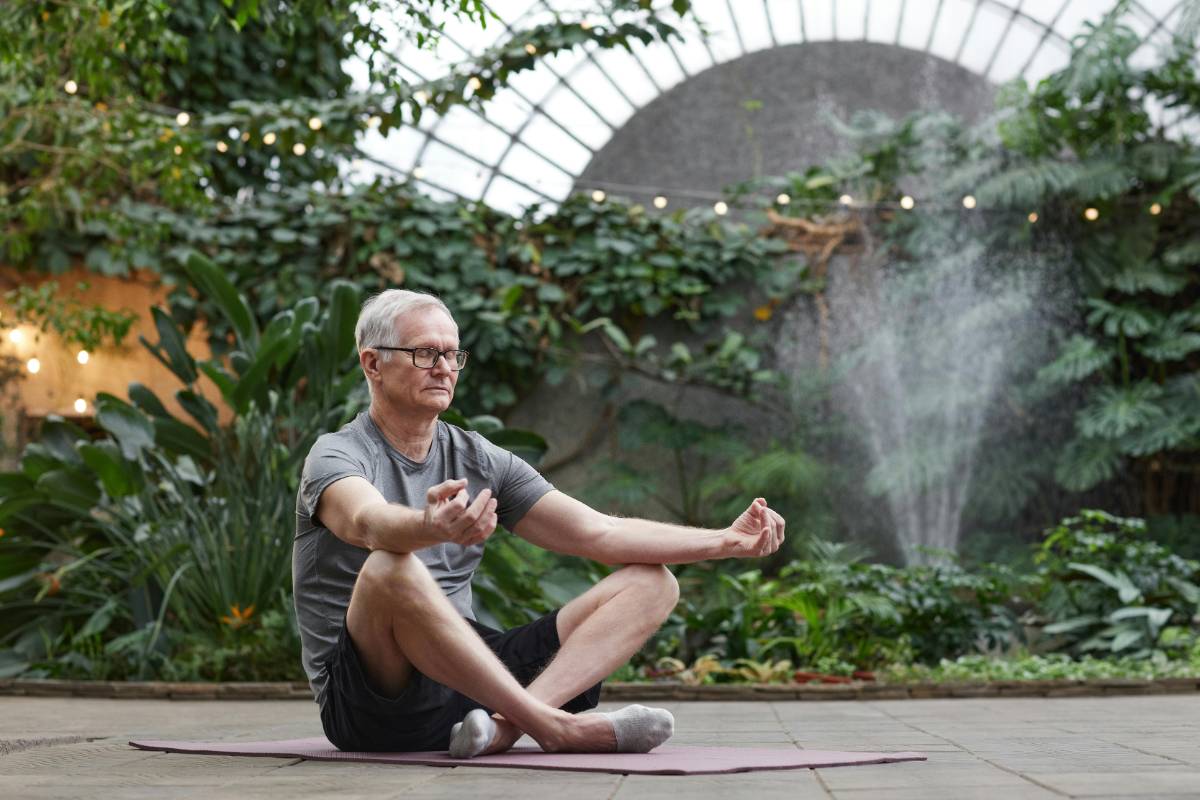
The Rise of Web3: Decentralized Internet on the Blockchain
6 min read | 02 Sep 2024

Blockchain and the Future of Jobs: How Automation Will Reshape the Workforce
5 min read | 01 Sep 2024

The Environmental Impact of Blockchain: Exploring Sustainable Solutions
7 min read | 31 Aug 2024
More Articles

Tokenomics: Understanding the Economics of Cryptocurrencies
5 min read | 26 Aug 2024

Decentralized Finance (DeFi): Redefining Financial Services
5 min read | 25 Aug 2024

Blockchain Scalability Solutions: Overcoming Limitations for Mass Adoption
5 min read | 24 Aug 2024

Privacy Coins: Enhancing Anonymity in Digital Transactions
6 min read | 23 Aug 2024